Measuring the mobile body
New border and surveillance technologies are being lauded for their accuracy and fairness. But how ethical can forced identification be? Late nineteenth-century enthusiasts of pinning down the ‘born criminal’ enlisted scientific advances to sinister ends. Might biometric data processing that registers migrants entering the EU risk a similar transgression of human rights today?
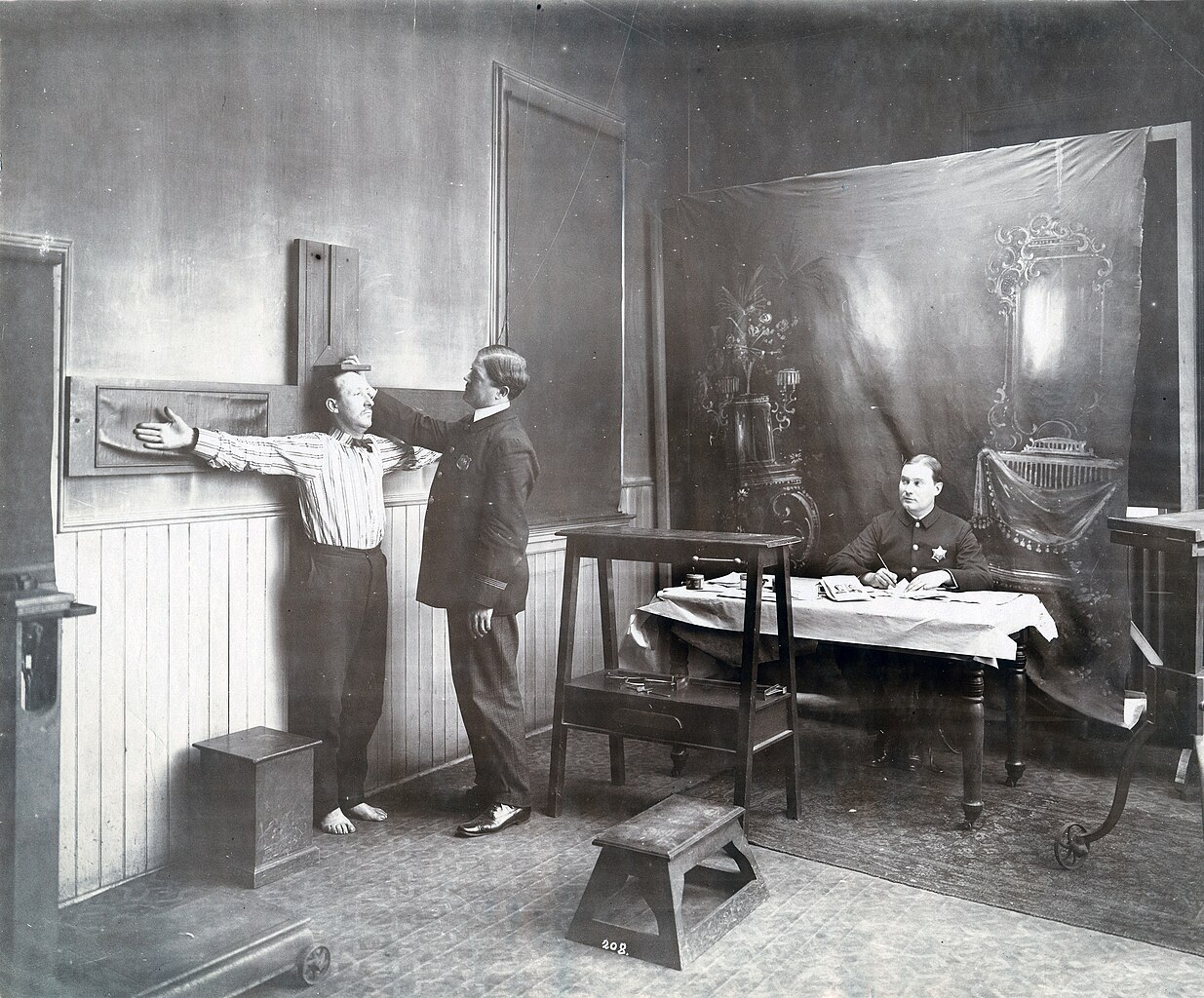
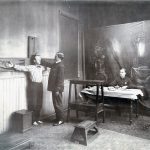
Europe’s high-tech arsenal of border technologies is often narrated as a futuristic tale of light, speed and computing power. Identification systems such as the Eurodac database store, process and compare the digitized fingerprints of migrants using near-infrared light, fibre optic cables and centralized servers. Drones patrol the skies with their unblinking optical sensors. And large volumes of data are fed to computer programmes that predict the next surge in arrivals.
News stories and NGO reports focusing on the high-tech nature of European borders abound. Each elicit how remote forms of surveillance, deterrence and control increasingly supplement and, in certain cases, supersede border fortifications. While this kind of research and advocacy is essential for holding the EU and tech developers to account for their role in driving asylum seekers towards lethal migration routes, it glosses over the long histories of these technologies and their established role in Western apparatuses of governance. This not only risks amplifying ‘AI hype’ among policymakers and developers, who hail these tools as a means both to create ‘smarter’ borders and to protect the human rights of migrants. More importantly, this kind of historical amnesia can misread the violence and exclusions enacted by technical ‘bias’, which is seemingly easily corrected by more accurate measurements or larger datasets. Instead, much of the harm incurred by these technologies should be understood as inherent in their design.
A catalogue of identification
The deployment of advanced technologies to control human mobility is anything but new. Picture an urban European police station in the late nineteenth century. If the municipality had adopted the latest identification technology, suspects would have been subjected to a complex measurement process. Taking down their measurements was a precise and highly specialized process, requiring a skilled and trained technician.
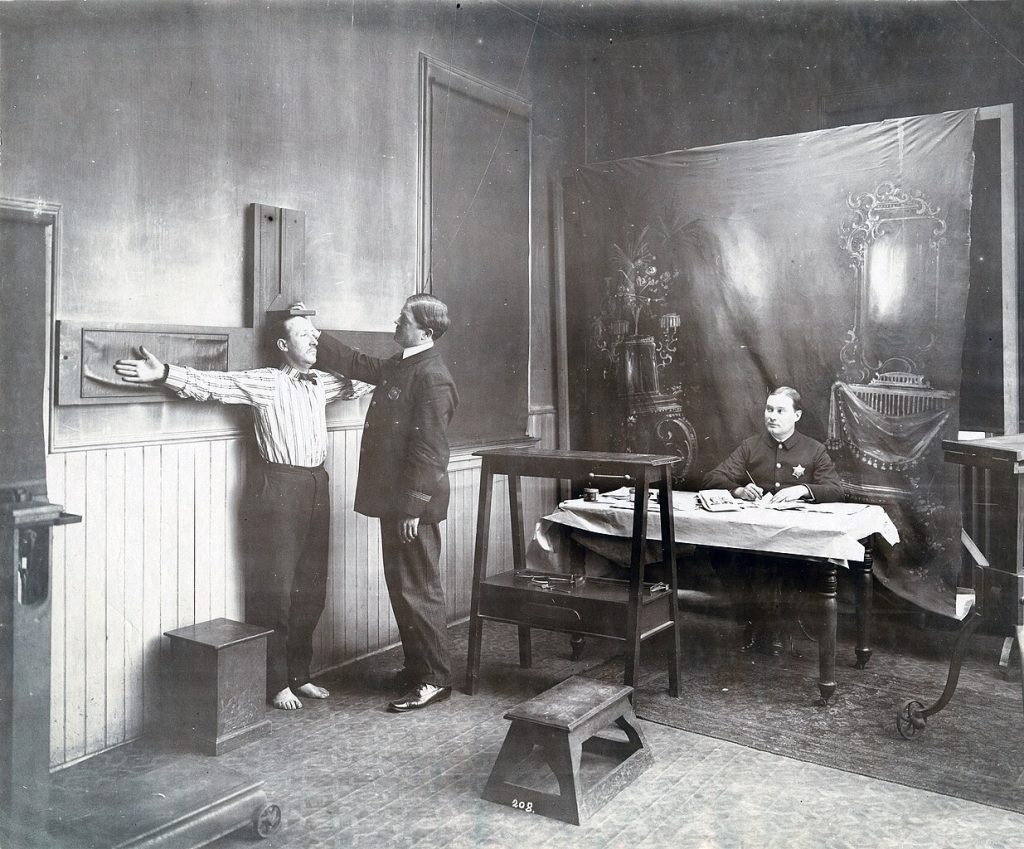
Bertillion measurements being taken in the Palace of Education at the 1904 World Fair. Image via Missouri History Museum, Wikimedia Commons
Consider these instructions for measuring an ear:
The operator brings the instrument’s fixed jaw to rest against the upper edge of the ear and immobilizes it, pressing his left thumb fairly firmly on the upper end of the instrument’s jaw, with the other fingers of the hand resting on the top of the skull. With the stem of the calliper parallel to the axis of the ear, he gently pushes the movable jaw until it touches the lower end of the lobe and, before reading the indicated number, makes sure that the pinna [external part of the ear] is in no way depressed by either jaw.
A. Bertillon, Instructons signalétiques, Melun, 1893, plate 16, p. 262.
This process may sound like a quaint if somewhat curious relic of the Fin de Siècle, but it is anything but. Bertillonage, the system of measurement, classification and archiving for criminal identification devised in the 1870s by the eponymous French police clerk, was a milestone in the history of surveillance and identification technology. Remarkably, its key tenets underwrite identification technologies to this day, from the database to biometrics and machine learning.
A close and historically established link exists between fears around the uncontrolled circulation of various ‘undesirables’ and technological innovation. Nineteenth century techniques, developed and refined to address problems around vagrancy, colonial governance, deviance, madness and criminality, are the foundations of today’s high-tech border surveillance apparatus. These techniques include quantification, which renders the human body as code, classification, and modern methods of indexing and archiving.
Modern invasive registration
Smart border systems employ advanced technologies to create ‘modern, effective and efficient’ borders. Accordingly, advanced technologies translate border processes such as identification, registration and mobility control into a purely technical procedure, seemingly rendering the process itself fairer and less fallible. Algorithmic precision is often portrayed as a means of avoiding unethical political biases and correcting human error.
As a researcher of the technoscientific underpinnings of the EU’s high-tech border apparatus, I am part of a team of researchers at the NOMIS-funded Elastic Borders project, University of Graz, Austria.
I recognize both the increasing elasticity of contemporary border practices, and the historically established methodology of its tools and practices.
See also: M. Maguire, ‘Biopower, Racialization and New Security Technology’, Social Identities, Vol. 18, No.5, 2012, pp. 593-607; K. Donnelly, ‘We Have Always Been Biased: Measuring the human body from anthropometry to the computational social sciences’, Public, Vol. 30, No. 60, 2020, pp. 20-33; A. Valdivia and M. Tazzioli, ‘Genealogies beyond Algorithmic Fairness: Making up racialized subjects’, in Proceedings of the 2023 ACM Conference on Fairness, Accountability, and Transparency, FAccT ’23, Association for Computing Machinery, 2023, pp. 840-50.
Take the Eurodac database, a cornerstone of EU border management, for example. Established in 2003, the index stores asylum seeker fingerprints as enforcement of the Dublin Regulation on first entry. If prints were taken in Greece, but the asylum seeker was later apprehended in Germany, they could face removal to Greece for processing their application.
Fingerprinting and enrolment in interoperable databases are also central tools utilized in recent approaches to migration management such as the Hotspot Approach, where the attribution of identity serves as a means to filter out ‘deserving’ from ‘undeserving’ migrants.
B. Ayata, K. Cupers, C. Pagano, A. Fyssa and D. Alaa, The Implementation of the EU Hotspot Approach in Greece and Italy: A comparative and interdisciplinary analysis (working paper), Swiss Network for International Studies, 2021, p. 36.
Over the years, both the type of data stored on Eurodac and its uses have expanded: its scope has been broadened to serve ‘wider migration purposes’, storing data not only on asylum seekers but also on irregular migrants to facilitate their deportation. A recently accepted proposal has added facial imagery and biographic information, including name, nationality and passport information, to fingerprinting. Furthermore, the minimum age of migrants whose data can be stored has been lowered from fourteen to six years old.
Since 2019 Eurodac has been ‘interoperable’ with a number of other EU databases storing information on wanted persons, foreign residents, visa holders and other persons of interest to criminal justice, immigration and asylum adminstrations, effectively linking criminal justice with migration whilst also vastly expanding access to this data. Eurodac plays a key role for European authorities, demonstrated by efforts to achieve a ‘100% fingerprinting rate’: the European Commission has pushed member states to enrol every newly arrived person in the database, using physical coercion and detention if necessary.
Marking criminality
While nation states have been collecting data on citizens for the purposes of taxation and military recruitment for centuries, its indexing, organization in databases and classification for particular governmental purposes – such as controlling the mobility of ‘undesirable’ populations – is a nineteenth-century invention. J.B. Rule, Private Lives and Public Surveillance, Allen Lane, 1973.
The French historian and philosopher Michel Foucault describes how, in the context of growing urbanization and industrialization, states became increasingly preoccupied with the question of ‘circulation’. Persons and goods, as well as pathogens, circulated further than they had in the early modern period.
Ibid., p. 91.
While states didn’t seek to suppress or control these movements entirely, they sought means to increase what was seen as ‘positive’ circulation and minimize ‘negative’ circulation. They deployed the novel tools of a positivist social science for this purpose: statistical approaches were used in the field of demography to track and regulate phenomena such as births, accidents, illness and deaths.
M. Foucault, Society Must be Defended. Lectures at the Collège de France, 1975-76, trans. D. Macey, Picador, 2003, p. 244.
The emerging managerial nation state addressed the problem of circulation by developing a very particular toolkit amassing detailed information about the population and developing standardized methods of storage and analysis.
One particularly vexing problem was the circulation of known criminals. In the nineteenth century, it was widely believed that if a person offended once, they would offend again. However, the systems available for criminal identification were woefully inadequate to the task.
As criminologist Simon Cole explains, identifying an unknown person requires a ‘truly unique body mark’. S. A. Cole, Suspect identities: A history of fingerprinting and criminal identification, Harvard University Press, 2001, p.12.
Yet before the advent of modern systems of identification, there were only two ways to do this: branding or personal recognition. While branding had been widely used in Europe and North America on convicts, prisoners and enslaved people, evolving ideas around criminality and punishment largely led to the abolition of physical marking in the early nineteenth century. The criminal record was established in its place: a written document cataloguing the convict’s name and a written description of their person, including identifying marks and scars.
However, identifying a suspect from a written description alone proved challenging. And the system was vulnerable to the use of aliases and different spellings of names: only a person known to their community could be identified with certainty. Early systems of criminal identification were fundamentally vulnerable to mobility. Ibid., pp. 18-9.
Notably, these problems have continued to haunt contemporary migration management, as databases often contain multiple entries for the same person resulting from different transliterations of names from Arabic to Roman alphabets.
The invention of photography in the 1840s did little to resolve the issue of criminal identification’s reliability. Not only was a photographic record still beholden to personal recognition but it also raised the question of archiving. Criminal records before Bertillonage were stored either as annual compendiums of crimes or alphabetical lists of offenders. While photographs provided a more accurate representation of the face, there was no way to archive them according to features. If one wanted to search the index for, say, a person with a prominent chin, there was no procedure for doing so. Photographs of convicts were sorted alphabetically according to the name provided by the offender, thereby suffering from the same weakness as other identification systems.
Datafication’s ancestor
Alphonse Bertillon was the first to solve this problem by combining systematic measurements of the human body with archiving and record keeping. The criminologist improved record retrieval by sorting entries numerically rather than alphabetically, creating an indexing system based entirely on anthropomorphic measurements. Index cards were organized according to a hierarchical classificatory system, with information first divided by sex, then head length, head breadth, middle finger length, and so forth. Each set of measurements was divided into groups based on a statistical assessment of their distribution across the population, with averages established by taking measurements from convicts. The Bertillon operator would take a suspect’s profile to the archive and look for a match through a process of elimination: first, excluding sex that didn’t match, then head lengths that didn’t match, and so forth. If a tentative match was found, this was confirmed with reference to bodily marks also listed on the card. Wherever this system was implemented, the recognition rates of ‘recidivists’ soared; Bertillon’s system soon spread across the globe. Ibid., pp. 34-45.
With Bertillon, another hallmark of contemporary border and surveillance technology entered the frame: quantification, or what is known as ‘datafication’ today. Bertillon not only measured prisoners’ height and head lengths but invented a method to translate distinctive features of the body into code. For instance, if a prisoner had a scar on their forearm, previous systems of criminal identification would have simply noted this in the file. By contrast, Bertillon measured their distance from a given reference point. These were then recorded in a standardized manner using an idiom of abbreviations and symbols that rendered these descriptions in abridged form. The resulting portrait parlé, or spoken portrait, transcribed the physical body into a ‘universal language’ of ‘words, numbers and coded abbreviations’. Ibid., p.48.
For the first time in history, a precise subject description could be telegraphed.
The translation of the body into code still underwrites contemporary methods of biometric identification. Fingerprint identification systems that were first trialled and rolled out in colonial India converted papillary ridge patterns into a code, which could then be compared to other codes generated in the same manner. Facial recognition technology produces schematic representations of the face and assigns numerical values to it, thereby allowing comparison and matching. Other forms of biometric ID like voice ID, iris scans, and gait recognition follow this same principle.
From taxonomy to machine learning
Besides quantification, classification – a key instrument of knowledge generation and governance for centuries – is another hallmark of modern and contemporary surveillance and identification technologies. As noted by many scholars from Foucault M. Foucault, The Order of Things. Routledge, 1975.
to Zygmunt Bauman
Z. Bauman, Modernity and the Holocaust, Blackwell Publishers, 1989.
and Denise Ferreira da Silva
D. Ferreira da Silva, Toward a Global Idea of Race, University of Minnesota Press, 2007.
, classification is a central tool of the European Enlightenment, evidenced most iconically by Carl Linnaeus’ taxonomy. In his graduated table, Linnaeus named, classified and hierarchically ordered the natural world from plants to insects to humans, dividing and subdividing each group according to shared characteristics. Classification and taxonomies are widely seen as an expression of the fundamental epistemological shifts from a theocentric to a rationalistic epistemology in the early modern era, which enabled scientific breakthroughs but were also tied to colonization and enslavement.
S. Wynter, ‘Unsettling the coloniality of being/power/truth/freedom: Towards the human, after man, its overrepresentation – an argument’, CR: The New Centennial Review, Vol. 3, No. 3, 2003, pp. 257-337.
In their book on the theme, Geoffrey Bowker and Susan Leigh Star underscore classification’s use as a powerful but often unrecognized instrument of political ordering: ‘Politically and socially charged agendas are often first presented as purely technical and they are difficult even to see. As layers of classification system become enfolded into a working infrastructure, the original political intervention becomes more and more firmly entrenched. In many cases, this leads to a naturalization of the political category, through a process of convergence. It becomes taken for granted.’
G. C. Bowker and S. L. Star, Sorting things out: Classification and its consequences, MIT press, 2000, p. 196.
Today, classification is central to machine learning, a subfield of artificial intelligence designed to discern patterns in large amounts of data. This allows it not only to categorize vast amounts of information but also to predict and classify new, previously unseen data. In other words, it applies learned knowledge to new situations. While research on machine learning began in the middle of the last century, it has come to unprecedented prominence recently with applications like ChatGPT.
Machine learning is also increasingly applied in border work. Rarely used as a stand-alone technology, it is widely deployed across existing technologies to augment and accelerate long-established forms of surveillance, identification and sorting. For instance, algorithmic prediction, which analyses large amounts of data including patterns of movement, social media posts, political conflict, natural disasters, and more, is increasingly replacing statistical migration modelling for the purpose of charting migratory patterns. The European Commission is currently funding research into algorithmic methods which would expand existing forms of risk analysis by drawing on wider data sources to identify novel forms of ‘risky’ conduct. Machine learning is also being either trialled or used in ‘lie detector’ border guards, dialect recognition, tracking and identification of suspicious vessels, facial recognition at the EU’s internal borders and behavioural analysis of inmates at Greek camps. As this wide range of applications illustrates, there would seem to be no border technology exempt from machine learning, whether assisted image analysis of drone footage or the vetting of asylum claims.
Classification lies at the core of machine learning – or at least the type of data-driven machine learning that has become dominant today. Individual data points are organized into categories and sub-categories, a process conducted either through supervised or unsupervised learning. In supervised learning, training data is labelled according to a predefined taxonomy. In practice, this usually means that humans assign labels to data such as ‘dog’ to an image of said dog. The machine learning model learns from this labelled dataset by identifying patterns that correlate with the labels. In unsupervised learning, the data is not labelled by humans. Instead, the algorithm independently identifies patterns and structures within the data. In other words, the algorithm classifies the data by creating its own clusters based on patterns inherent in the dataset. It creates its own taxonomy of categories, which may or may not align with human-created systems.
The supposed criminal type
As the AI and border scholar Louise Amoore points out, casting algorithmic clusters as a representation of inherent, ‘natural’ patterns from data is an ‘extraordinarily powerful political proposition’ as it ‘offers the promise of a neutral, objective and value-free making and bordering of political community’. L. Amoore, ‘The deep border’, Political Geography, 2001, 102547.
The idea of the algorithmic cluster as a ‘natural community’ comprises a significant racializing move: forms of conduct associated with irregular migration are consequently labelled as ‘risky’. As these clusters are formed without reference to pre-defined criteria, such as ‘classic’ proxies for race like nationality or religion, they are difficult to challenge with existing concepts like protected characteristics or bias.
Ibid.
For instance, a migrant might be identified as a security risk by a machine learning algorithm based on an opaque correlation between travel itineraries, social media posts, personal and professional networks, and weather patterns.
The creation of categories according to inherent attributes echoes and extends to other nineteenth-century practices: namely, a range of scientific endeavours using measurement and statistics to identify regularities and patterns that would point to criminal behaviour. Like unsupervised machine learning, the fields of craniometry, phrenology and criminal anthropology systematically accumulated data on human subjects to glean patterns that could be sorted into categories of criminality.
For instance, phrenologists like Franz Joseph Gall linked specific personality traits to the prominence of regions of the skull. In the related field of physiognomy, figures like the Swiss pastor Johann Kaspar Lavater undertook a systematic study of facial features as a guide to criminal behaviour. Fuelled by the development of photography, studies investigating signs of criminality in the face gained traction, with convicts and inmates of asylums repeatedly subjected to such ‘studies’. The composite photographs of Frances Galton, the founder of the eugenics movement and a pioneer of fingerprint identification, are a case in point: images of convicts were superimposed onto one another to glean regularities as physical markers of criminality. Galton conducted a similar study on Jewish school boys, searching for racial markers of Jewishness.
Criminal anthropology consolidated these approaches into a coherent attempt to subject the criminal body to scientific scrutiny. Under the leadership of the Italian psychiatrist and anthropologist Cesare Lombroso, criminal anthropologists used a wide range of anthropomorphic tools of measurement, from Bertillon’s precise measurements of limbs to craniometric skull measurements, mapping facial features, and noting distinctive marks like scars and tattoos. On this basis, they enumerated a list of so-called ‘stigmata’ or physical regularities found in the body of the ‘born criminal’ While this notion is widely discredited today, the underlying method of classification based on massed data characteristics still exists.
Trusting the conclusions drawn from quantitative analysis of facial features remains a strong allure. A 2016 paper claimed it had successfully trained a deep neural network algorithm to predict criminality based on head shots from drivers licenses, while a 2018 study made similar claims about reading sexual orientation from dating site photos.
When engaging critically with these systems, it is imperative to remain mindful of the larger political project they are deployed to uphold. As AI scholar Kate Crawford writes: ‘Correlating cranial morphology with intelligence and claims to legal rights acts as a technical alibi for colonialism and slavery. While there is a tendency to focus on the errors in skull measurements and how to correct for them, the far greater error is in the underlying worldview that animated this methodology. The aim, then, should be not to call for more accurate or “fair” skull measurements to shore up racist models of intelligence but to condemn the approach altogether.’ K. Crawford, The Atlas of AI: Power, Politics, and the Planetary Costs of Artificial Intelligence, Yale University Press, 2021, pp. 126-7.
Put differently, techniques of classification and quantification cannot be divorced from the socio-political contexts they are tasked to verify and vouch for. To rephrase International Relations scholar Robert Cox, classification and quantification are always for someone, and for some purpose.
R. W. Cox, ‘Social Forces, States and World Orders: Beyond International Relations Theory’, Millennium, Vol. 10, No. 2, 1981, pp. 126–155.
Yet, as Science and Technology Studies scholar Helga Nowotny cautions, if we ‘trust’ the results of algorithmic prediction as fundamentally true, we misunderstand the logic of deep neural networks. These networks ‘can only detect regularities and identify patterns based on data that comes from the past. No causal reasoning is involved, nor does an AI pretend that it is.’ H. Nowotny, In AI We Trust: Power, Illusion and Control of Predictive Algorithms. Polity, 2021, p. 22.
While these machines may produce ‘practical and measurable predictions’, they have no sense of cause and effect – in short, they have no ‘understanding’ in the human sense. Ibid.
Furthermore, an overreliance on algorithms nudges us toward determinism, aligning our behaviour with machinic prediction in lieu of alternative paths. This is a problem in political cultures premised on accountability. If we wish to learn from the past to build better futures, we cannot rely on the predictive outputs of a machine learning model.
AI déjà-vu
There are many threads besides the shared and continued reliance on quantification and classification one could pull on to explore the entangled history of surveillance and identification technologies from the nineteenth century to the present. Marginalized, surplus populations like convicts and colonized people have long been used as ‘technological testing grounds’ to hone classificatory systems and train algorithms. A fear of uncontrolled human mobility continues to be leveraged as a driver for research and development, with tech, in turn, deployed to fix problems it has itself created. And positivistic social scientific methods remain instrumental to the task of translating roaring multiplicities into neat, numerical values.
Instead of falling for AI hype, we might instead attune ourselves to a sense of déjà-vu: the unsettling feeling that we’ve seen all this before. This way, we might better resist the fantastical claims made by corporate and border actors, and begin uncoupling technologies from global projects of domination.
This article is based on research carried out during the project ‘Elastic Borders: Rethinking the Borders of the 21st Century’ based at the University of Graz, funded by the NOMIS foundation.